Research
Among all medical data, genomes play a very specific role. A genome is a text - digital by nature. It is almost perfectly measured and it is cheap to obtain. The inherited genome, or germline genome, is a constant throughout the life of an individual. It can be a cause but not a consequence of diseases. Causal predictions can be experimentally validated by genome edition technologies. Furthermore, the genome encodes RNAs and proteins, offering a valuable entry point to understanding cellular mechanisms and designing pharmaceutical interventions.
So far, however, much of the human genetic sequence is not understood. Specifically, the regulatory code, those genetic instructions determining when, where, and how much RNAs and proteins shall be available, is poorly deciphered. Consequently, most of the variants associated with common diseases, which are non-coding, remain poorly interpreted. Also, no genetic diagnosis can be provided for the majority of patients with rare diseases that show no obvious disease-causing coding variant.
Our goal is an improved understanding of the genetic basis of gene regulation and its implication in diseases. To this end, we employ statistical modeling of omic data and work in close collaboration with experimentalists. This research program is articulated around three axes: Measure, model, and apply.
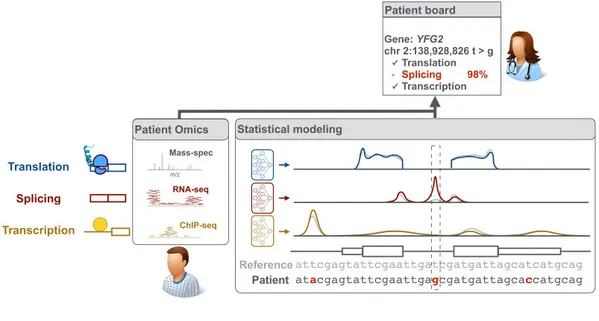
Axis 1 - Measure: Improved measurements of gene expression
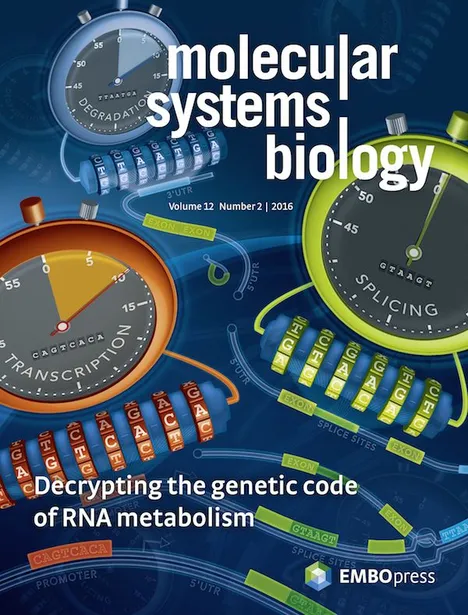
We collaborate with experimentalists to precisely quantify genome-wide every step of gene expression from RNA synthesis to protein abundance. This includes designing novel experimental approaches [1,2], or pushing the analytic boundaries of high-throughput assays based on next-generation sequencing or mass spectrometry [3].
Axis 2 - Model: Deciphering the regulatory code
Our studies not only help in deciphering the regulatory code at all steps of gene expression from transcription, splicing, RNA degradation to protein translation and degradation [4-10] but also in delineating fundamental gene regulatory mechanisms [11,12]. At the heart of this endeavor lies the development of innovative machine learning algorithms, notably using deep learning, which allows us to flexibly model the complexity of the regulatory code while leveraging very rich omics datasets [9,13].
In this context, we are collaborating with the labs of Kevin Verstrepen and Vicent Pelechano on a 6-year (2024-2030) ERC Synergy grant called EPIC to unravel the complete regulatory code of yeast See more here.
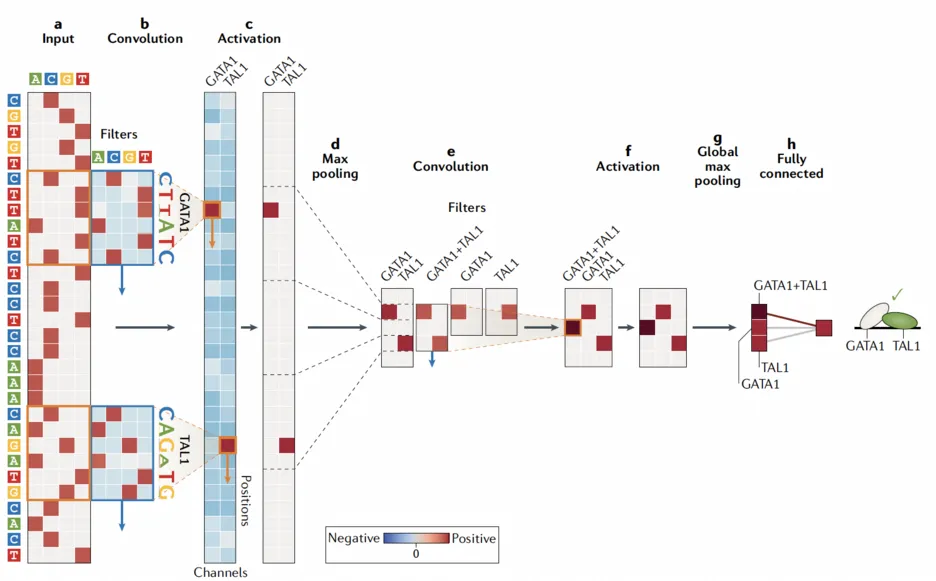
Axis 3 - Apply: Applications for genome-based medicine
Determining the genetic cause of rare disorders is crucial for the affected families, enabling genetic testing among relatives and providing a rationale for therapies. However, for most of the rare disease patients undergoing DNA sequencing, which variant is pathogenic remains unclear. Together with the Prokisch group at the Institute of human genetics in Munich, we have pioneered an effective approach in which we sequence not only DNA, but also RNA, of patients suspected to be affected with a genetic disorder [14]. This led to a boost in diagnosis, pinpointing the disease-causing gene for 15% of unsolved cases for some disease entities [15]. We are developing this approach further, using improved algorithms [16,17], larger datasets, and more data modalities (proteomics, phenotypic descriptors, etc.). We also extend the spectrum of applications of our technologies to cancer and common disorders.

Compute infrastructure
Our lab operates a secure computing infrastructure for the storage and analysis of human genomics data. Co-financed by the DFG (INST 95/1655-1 FUGG and INST 95/1863-1 FUGG), the system comprises over 3,000 CPUs, 60 GPUs, 14 TB RAM, and 2.5 PB of encrypted storage. It supports several national and international research initiatives, including Solve-RD, ERDERA, TRR267, and the ERC-funded project EPIC. Inquiries may be directed to assistant-gagneurlab@in.tum.de.
Community
We are contributing to the genomics research community with the development of open-source scientific software (R/Bioconductor or python), including the repository of trained models for genomics Kipoi [18]. We are proud members of the national infrastructure German Human Genome-Phenome Archive and collaborate with multiple groups on rare disease research, notably the European project Solve-RD.
Spin-off
Three lab members, Vicente Yépez, Christian Mertes and Felix Brechtmann, launched the TUM spin-off start-up OmicsDiscoveries GmbH in November 2024 leveraging the tools and methodologies developed in the lab for the analysis of RNA-seq data in rare diseases.
OmicsDiscoveries supports clinicians in studying rare disease patients by providing automated reports and an analysis platform through an advanced ML-based analysis of DNA, RNA, and clinical data. See also their LinkedIn profile.
References
1. Schwalb et al., TT-seq maps the human transient transcriptome, Science, 2016
2. Rojas Ringeling et al. Partitioning RNAs by length improves transcriptome reconstruction from short-read RNA-seq data. Nature Biotechnology, 2022
3. Klaproth-Andrade et al. Deep learning-driven fragment ion series classification enables highly precise and sensitive de novo peptide sequencing. bioRxiv, 2023
4. Eser et al. Determinants of RNA metabolism in the Schizosaccharomyces pombe genome, Molecular Systems Biology, 2016
5. Cheng et al. Cis-regulatory elements explain most of the mRNA stability variation across genes in yeast, RNA, 2017
6. Cheng et al. MMSplice: modular modeling improves the predictions of genetic variant effects on splicing, Genome Biology, 2019
7. Eraslan et al. Quantification and discovery of sequence determinants of protein per mRNA amount in 29 human tissues, Molecular Systems Biology, 2019
8. Wachutka et al., Global donor and acceptor splicing site kinetics in human cells, eLife, 2019
9. Avsec et al. Base-resolution models of transcription factor binding reveal soft motif syntax. Nature Genetics, 2021
10. Wagner et al. Aberrant splicing prediction across human tissues. Nature genetics, in press
11. Bader et al. Negative feedback buffers effects of regulatory variants, Molecular Systems Biology, 2015
12. Tomaz da Silva et al. Cellular energy regulates mRNA translation and degradation in a codon-specific manner. bioRxiv, 2023
13. Gankin et al. Species-aware DNA language modeling. bioRxiv 2023
14. Kremer et al. Genetic diagnosis of Mendelian disorders via RNA sequencing, Nature Communications, 2017
15. Yépez et al. Clinical implementation of RNA sequencing for Mendelian disease diagnostics. Genome Medicine, 2022
16. Brechtmann et al. OUTRIDER: A statistical method for detecting aberrantly expressed genes in RNA sequencing data, AJHG, 2018
17. Mertes et al. Detection of aberrant splicing events in RNA-Seq data with FRASER. Nature Communications, 2021
18. Avsec et al. The Kipoi repository accelerates community exchange and reuse of predictive models for genomics, Nature Biotechnology, 2019