ThoraXAI - Trustworthy and explainable AI for Thorax Diagnostics
Contact Persons
Matthias Keicher (matthias.keicher(at)tum.de)
Abstract
The automatic diagnosis of thoracic images has gained importance, especially after COVID-19. However, a lack of trustworthiness presents a significant obstacle in their implementation in clinical practice. Among these, the reliability and explainability of AI models are central, as they suffer from epistemic uncertainty in domain shifts, or rely on incorrect features and cannot generalize. With the help of explainability methods, we can understand the features these models rely on, their decision-making mechanism, and the source of their uncertainty, and take measures to improve the models in terms of reliability. In ThoraXAI, Siemens Healthineers is researching with TUM and Klinikum Rechts der Isar the intersection of uncertainty and explainability of AI models, developing approaches that enable reliable automatic evaluation of X-ray and CT images.
Keywords: Upper body CT images, chest X-ray, trustworthiness, vision-language models, explainability, semantic features, uncertainty, decision-support
Research Partners
This project is funded by the Bavarian Ministry of Economic Affairs, Regional Development and Energy (StMWi) as part of the Förderlinie "Digitalisierung" program under the grant agreement DIK-2302-0002.
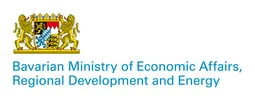