Are Defenses for Graph Neural Networks Robust?
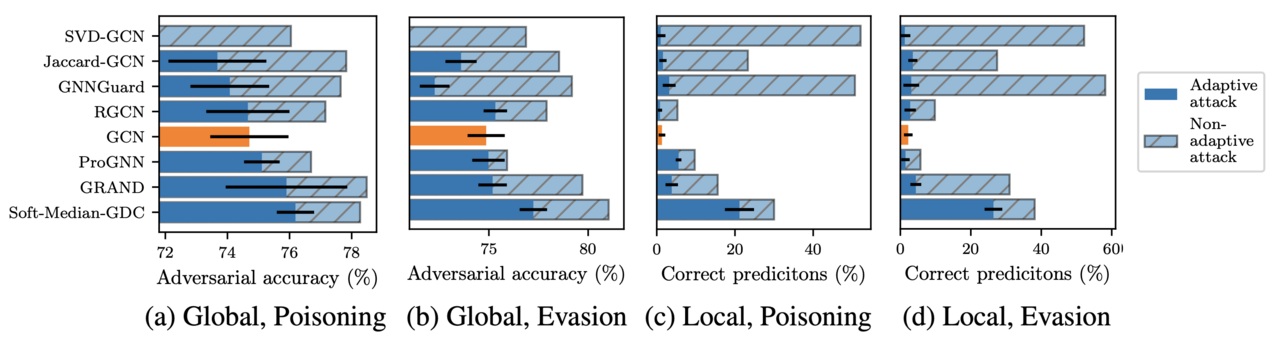
This page links to additional material for our paper
Are Defenses for Graph Neural Networks Robust?
by Felix Mujkanovic*, Simon Geisler*, Stephan Günnemann, and Aleksandar Bojchevski
* equal contribution
Published at the Neural Information Processing Systems (NeurIPS) 2022.
Links
[Paper (OpenReview) | Code (GitHub) | Video (YouTube) ]
Abstract
A cursory reading of the literature suggests that we have made a lot of progress in designing effective adversarial defenses for Graph Neural Networks (GNNs). Yet, the standard methodology has a serious flaw – virtually all of the defenses are evaluated against non-adaptive attacks leading to overly optimistic robustness estimates. We perform a thorough robustness analysis of 7 of the most popular defenses spanning the entire spectrum of strategies, i.e., aimed at improving the graph, the architecture, or the training. The results are sobering – most defenses show no or only marginal improvement compared to an undefended baseline. We advocate using custom adaptive attacks as a gold standard and we outline the lessons we learned from successfully designing such attacks. Moreover, our diverse collection of perturbed graphs forms a (black-box) unit test offering a first glance at a model's robustness.
Cite
Please cite our paper if you use the method in your own work:
@inproceedings{mujkanovic2022_are_defenses_for_gnns_robust,
title = {Are Defenses for Graph Neural Networks Robust?},
author = {Mujkanovic, Felix and Geisler, Simon and G\"unnemann, Stephan and Bojchevski, Aleksandar},
booktitle={Neural Information Processing Systems, {NeurIPS}},
year = {2022}
}