We combine ideas from Scientific Computing and Machine Learning to create understandable, robust, and effective data-driven algorithms.
These projects are currently running:
- Harmonic AI based on Linear Operators
- Scientific Machine Learning Focus Group (IAS)
- GNI project DeepMonitor
- GNI project FORWARD
- AutoMD-AI: Surrogate models for molecular dynamics
Harmonic Artificial Intelligence based on Linear Operators
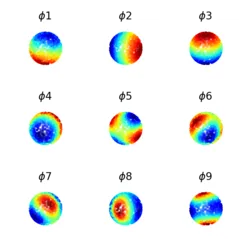
https://gepris.dfg.de/gepris/projekt/468830823?language=en
Emmy Noether group
Simulating complex, highly interconnected systems such as the climate, biology, or society typically involve methods from the "traditional" field of scientific computing. These methods are usually reliable and explainable through their foundations in rigorous mathematics. Examples are efficient space discretization schemes such as sparse grids and scalable, parallel solvers for partial differential equations. Unfortunately, most of them are not immediately applicable to the extremely high-dimensional, heterogeneous, and scattered data where neural networks are usually used. Those methods employed in the AI community, on the other hand, are typically not reliable or explainable in the traditional sense, and pose problems illustrated through adversarial examples and brittle generalization results. Toward the goal of explainable, reliable, and efficient AI, we connect the two worlds of scientific HPC and deep learning, forming the concept of "Harmonic AI". Specifically, we combine linear operator theory and deep learning methods through harmonic analysis. The benefit of a link between AI and linear operators is bi-directional. Inference, classification, and training of neural networks will be understood mostly in terms of linear algebra. This will open the field to much more mathematical rigor and enable more mathematicians to work on AI methods. Simultaneously, applied AI researchers obtain reliable methods that can currently only be found for problems outside the field, such as Finite Element Methods or iterative Newton-Raphson solvers.The first three years of the project are devoted to explainability of AI by bridging the gap to rigorous mathematics: Leveraging the common principles between the Laplace operator, Gaussian processes, and neural networks, Harmonic AI will connect AI and linear algebra. This will allow me to explain unsupervised data representation algorithms. The second core objective in this first phase is to bridge the theory between the linear Koopman operator and deep neural networks. We bring ideas from dynamical systems theory to the AI community, to explain the layered neural networks and stochastic optimization algorithms in terms of dynamical evolution operators.The core objective in the second phase (years four to six) is to devise robust and reliable numerical algorithms harnessing the connection between linear operators and neural networks from phase A. The algorithms will be integrated in an HPC environment. We consistently release free and open source software for Harmonic AI to make its algorithms available to a broad research community. Throughout the project, to disseminate, demonstrate, and test the new methods in a proof of concept, we collaborate with the simulation groups from C. Mendl studying quantum dynamics and from G. Köster studying human crowds.
Scientific Machine Learning Focus Group (IAS)
The Focus Group “Scientific Machine Learning” involves Hans Fischer Senior Fellow Prof. Wil Schilders (Eindhoven University of Technology) and his host Prof. Hans-Joachim Bungartz (Chair of Scientific Computing in Computer Science –SCCS, TUM Department of Informatics).
The PhD candidate Chinmay Datar is part of the group of Physics-Enhanced Machine Learning.
GNI project DeepMonitor
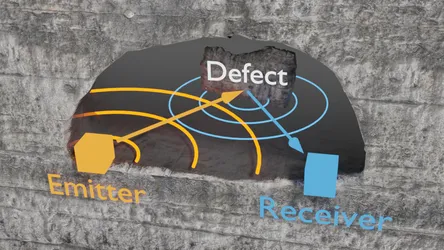
https://www.mdsi.tum.de/en/gni/gni-funded-projects/deepmonitor/
Structural flaws, especially when hidden, can compromise the integrity and safety of civil engineering constructions, such as buildings, bridges, and other infrastructure. Thus, non-invasive detection of hidden structural defects is a crucial task in civil engineering. The goal of the project is to develop a highly effective, data-driven framework that can detect structural defects in a computationally efficient manner. This framework will leverage advanced artificial intelligence (AI) and data science techniques to create a robust, efficient, and scalable solution for defect detection that can be used in practical civil engineering scenarios, enhancing structural safety and maintenance.
GNI project FORWARD
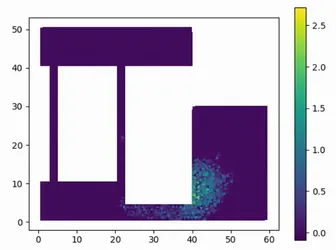
https://www.mdsi.tum.de/en/gni/gni-funded-projects/forward/
Project FORWARD aims to perform pedestrian flow prediction of in indoor and outdoor spaces, informing architects and engineers while designing buildings and infrastructure facilities. Specifically, the goal of the project is to compare and evaluate machine learning models in terms of accuracy and efficiency on the task of crowd modeling. The considered machine learning methods come from the class of deep neural networks as well as Koopman operator approaches from the scope of scientific machine learning.
AutoMD-AI: Surrogate models for molecular dynamics
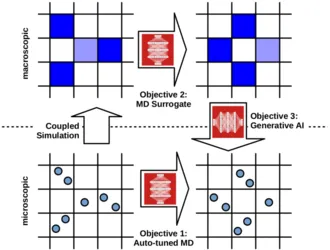
https://www.softwaresysteme.dlr-pt.de/de/machine-learning-modelle.php
In AutoMD-AI, the partners TUM and UHH develop ML-based methods to improve the efficiency and robustness of MD and coupled multiscale—so-called molecular-continuum—simulations on high performance computing (HPC) systems. The work focuses on three overarching objectives. Firstly, flexible machine learning (ML) algorithms are developed that fine-tune performance-critical components of MD simulations such as data structures, parallelization schemes, and algorithmic parameters. This adaptive tuning leads to scenario-dependent choices of algorithmic configurations, that can be adapted over time and in different regions of the MD system. Secondly, leveraging the "equation-free" framework, the microscopic (MD) simulation in coupled molecular-continuum simulations is accelerated by incorporating robust data-driven surrogate models on the macroscopic scale. A key challenge lies in determining when to switch between the surrogate model and the microscopic (MD) simulation code to ensure the reliability and robustness of the simulation results. This dynamic switching mechanism prevents the surrogate model from being applied in areas where its predictions are different from the original microscopic code, maintaining accuracy and trustworthiness. Thirdly, we employ generative ML algorithms to construct initial conditions on the microscopic scale for molecules, based on macroscopic criteria such as local density and flow velocity. This approach enables the efficient creation of thermodynamically accurate configurations without the need for extensive equilibration runs, which is a current challenge in the field. This also accelerates the dynamic switching from surrogate to MD in the molecular-continuum simulation.